In August, we published a broad overview of the data science for good landscape that captured what different initiatives were doing to advance the use of data in the social sector. One family of initiatives, Accelerating Data and Computing for Social Impact, focused on creating data science solutions for the social sector to solve more high-impact problems with this technology. Every initiative in this family either creates solutions themselves or reduces the barriers to creating data science solutions for the social sector.
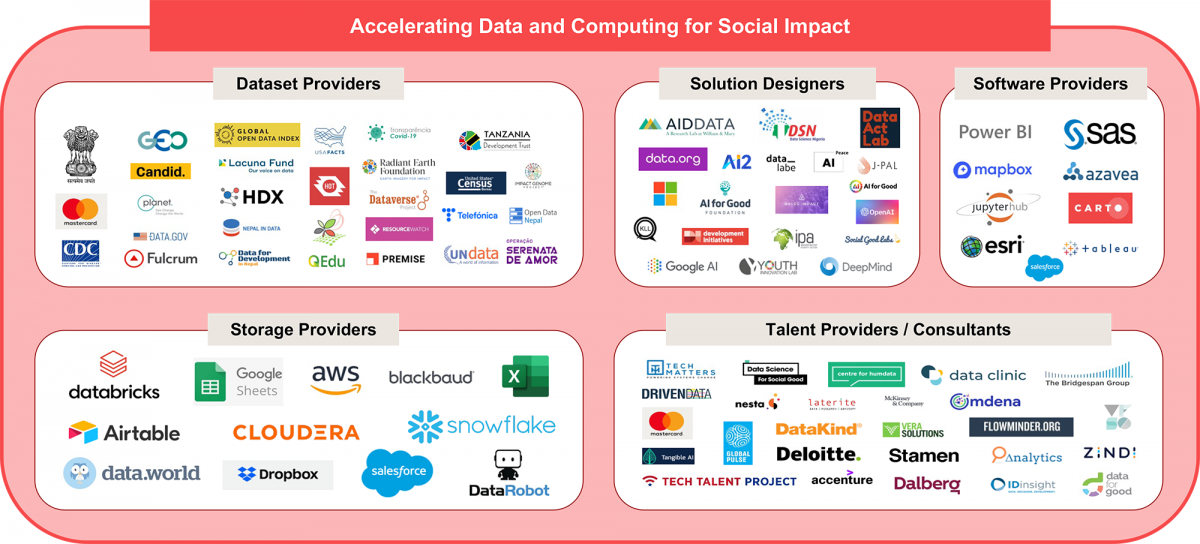
About the Author
Jake Porway loves seeing the good values in bad data. As a frustrated corporate data scientist, Porway co-founded DataKind, a nonprofit that harnesses the power of Data Science and AI in the service of humanity by providing pro bono data science services to mission-driven organizations.
Read moreAt first glance, the graphic is a plethora of services (and our forthcoming map has even more that we’ve recently added): Humanitarian Data Exchange provides hundreds of free humanitarian datasets, Amazon provides millions of free storage credits, Delta Analytics and DataKind provide pro bono data scientists by the droves. It’s exciting to see so much activity and so many pro bono and low bono services for the sector!
This configuration might work if an organization wants one specific, constrained problem solved, like getting storage for a dataset. However, any meaningful data project is often far more complex than a one-off solution. Imagine if you wanted to do a home improvement project and the only way to do it was to individually find different people to help you plan it, zone it, sell you the wood, sell you the nails, give you the tools, build it for you (or train you to do it), and paint and varnish it. Building that way is laborious and exhausting, so we’ve developed markets where you can simply hire a single contractor to manage all of that for you. No such market exists in the social sector, or at least not in a mature form, so nonprofits instead beg from organization to organization for whatever services they might have available, piecing them together into whatever they can. Nonprofits seeking to become digitally native have few options to do so holistically, and must instead build their digital capacity like a popsicle stick house – one brittle stick at a time, borrowed from whoever is offering.
This lack of clarity and a mature market contributes to the significant lag in the adoption of data science for social impact. Below we explore these challenges and outline several potential approaches to mitigate them — and move forward.
It Takes a Village (Unfortunately)
Take for example a project that I worked on when I was at DataKind. An educational non-profit – let’s call them Inspire Kids – wanted to analyze their data to understand what factors contributed to graduation rates. That sounds like a one-off project that an analyst could do if Inspire Kids could just give them their data. However, it took a coordinated effort of four different organizations, brought together largely by word of mouth. Early on it was clear the data wasn’t in a state to learn from, so an engineering firm was brought in to build a database system that brought the right data together and kept it clean. A major tech company was approached for partnership to provide free storage and computing power to keep the data pipeline running. Next, data scientists and analysts from DataKind did an analysis on the data to find correlations between student data and their outcomes. The model seemed to work well, but it would need to be re-run regularly to make sure it was up-to-date. A fourth team was needed to bring the solution to production, and they were only able to do the project because the major tech company that was providing the storage was able to call in a favor. That’s as many partners as I knew about, but I would suspect one would also need on-call capacity to help fix the tool if it broke, and trainers to help new staff understand how to use the tool, at a minimum. This hodgepodge way of combining services is often the norm in the social sector and causes huge amounts of friction. It also requires strong project management coordination, which many nonprofits may not have capacity for in-house.
What We Mean By Services
Of course, just saying these initiatives provide “data services” is a bit incomplete. Some provide training while others provide storage. The problem above remains, but let’s make it a little more nuanced by describing the types of services one might want and where our current landscape is lacking. Let’s use the house construction analogy again:
- You need repairs: If your toilet breaks or your lights go out, you know to call a plumber or an electrician to come repair your house. That’s the closest to a one-off project in this analogy you can get. Those situations arise in the data world too – you may need a database installed or a model updated – but the process for getting it done is far more painstaking. The first pain point is that it’s difficult to know what kind of help you need. We have learned enough in housing to know that issues with our pipes need someone called a “plumber”, but we don’t have that ease in the data world. Instead we have “data analysts”, “data scientists”, “technologists”, “coders”, and more. We might have a harder time discerning who to call if our service people in the house analogy were called “house fixer”, “repair engineer”, and “wood and water specialist”. Moreover, even if you can identify the type of help you need, there is no simple directory for finding pro bono data engineers the way there are phonebooks or directories of plumbers and electricians.
- You need a major project constructed: If you want to build something new on your house that you don’t have skills to do yourself, you’ll need to hire a contractor. The type of contractor you need might vary based on whether you’re adding on a deck or building an entirely new house from scratch, but nevertheless you’re likely going to interface with one or a few main contractors who will design, manage, and build the project for you. In the data science world, we do not have such central actors, or at least not many of them. It is often on the nonprofit to first determine what it would take to build their data science project and then request help on each part piecemeal, or to bounce from project design service to data collection service to data storage service, etc. There are a few one-stop shops that provide the whole service, but like the situation above, there is no easy way to find them. I had to look to the landscape to find a few, and the best I can do is think of Accenture (who still charges quite a bit) or a combo of a few other services.
- You want to learn to do repairs or construction yourself: Of course, you don’t always need to call for outside help. Many folks choose to train themselves to fix their own house, be that as small as repairing a hole in a screen to building a new deck yourself. In that case, you may want to subscribe to services to learn to do this yourself, ranging from YouTube videos walking you step-by-step through a project to full courses in electrical wiring or HVAC installation. You can find a similar analogy in the data world by looking at the many nonprofits who want to learn how to understand or build data solutions themselves. Many services exist to train folks in using data, but issues similar to the ones above persist. Without a clear sense of what types of data skills you need, it can be difficult to know what course to sign up for. Executives who just want an understanding of data science are daunted by Google searches returning courses on linear algebra and programming. People who want to analyze and visualize data for their funders struggle to navigate data artistry tutorials and statistical modeling programs. We don’t have enough clarity about the flavors of “data-driven” that we need to be to solve our problems, so it becomes difficult to discern which training initiatives will help us. Once we do, we’re stuck again with the problem of not having a directory outside of Google or word of mouth to find the best fit for us.
Moving Toward a More Coherent Approach to Data Services
So given the problems above, what can we do to ensure these data services are more comprehensible, accessible, and useful? Here are a few proposals that we believe would help the sector overall
- A directory of nonprofit data services in one single location. The landscape we built is a first stab at categorizing some of these services, but it’s currently too high level. It is useful for differentiating different types of activities, but a nonprofit seeking services would need more specifics about the types of data services available, their costs, time needed, and metrics to compare them. There is some early work being done to build this “yellowpages of digital services” by some of our partners that we’re excited about, and we would love to hear about any other similar efforts underway. A directory of services that allows nonprofits to find services tailored to their needs would be a big step forward.
- Clarity on data science outcomes. A recurring theme in the problems above is that it’s very difficult for individuals or organizations to identify the type of data and computing need they have. We have not established clear language in data for its outcomes, the way that “plumbing” is a stand-in for “water and pipe problems” or “electrician” is a stand-in for “electricity and wiring problems”. Some do exist in our field – technologists will likely know the differences between what a database engineer and a front end developer can do – but they are not consistent across organizations and they are not easily understandable by the layperson. Here at data.org, we have started to develop guides that describe what data can be used for axiomatically, based on its outcomes and not the technical activities around it, that we hope will help. We believe more people using clear language about the outcomes needed will get us closer to differentiating between the types of services a nonprofit needs.
- Clarity about what constitutes data maturity. Currently we talk about nonprofits becoming “data-driven” and “digitally native” as if there is one clear, single idea of what that looks like. Like the analogy above hopefully makes clear, there is no such thing as a singular “data-driven nonprofit”, any more than there is a singular “handy person”. Depending on your skill level, budget, and personal desires, you may want to solve a problem yourself or hire someone to solve it for you. Having different visions of what “data-driven” can look like could help nonprofits assess where they should enlist help in training vs. doing. At data.org, we are working on some different data maturity models that could advance this conversation.
- Coordinating entities. Even with the clarity above, it is likely that meaningfully difficult projects will still need multiple skills involved, just as building a house requires many different specialties. At present there are very few entities who will run a data project soup-to-nuts for nonprofits, and even fewer who will help coordinate partners to make it happen. The closest I’ve seen in my experience comes out of the multiparty grant proposals put forth by nonprofits who are already in partnership. That type of partnership is a luxury for those nonprofits already networked enough to have those connections and often the brand appeal and connections to then win the grant. We need a more egalitarian model, perhaps in the form of raising visibility of nonprofits tackling similar problems, or of foundations funding long-term consultancies or labs that provide guidance, coordination, and support to nonprofits from a centralized location.
Those are some of the initial reactions we had in looking at the data services landscape, but we want to hear yours. Which observations did we miss? Which did you disagree with? We believe there is huge potential for our field to create a data-driven social sector, but only if we all roll up our sleeves and figure out what’s missing together. We hope to make this space more coherent with your help!
About the Author
Jake Porway loves seeing the good values in bad data. As a frustrated corporate data scientist, Porway co-founded DataKind, a nonprofit that harnesses the power of Data Science and AI in the service of humanity by providing pro bono data science services to mission-driven organizations.
Read moredata.org In Your Inbox
Do you like this post?
Sign up for our newsletter and we’ll send you more content like this every month.
By submitting your information and clicking “Submit”, you agree to the data.org Privacy Policy and Terms and Conditions, and to receive email communications from data.org.